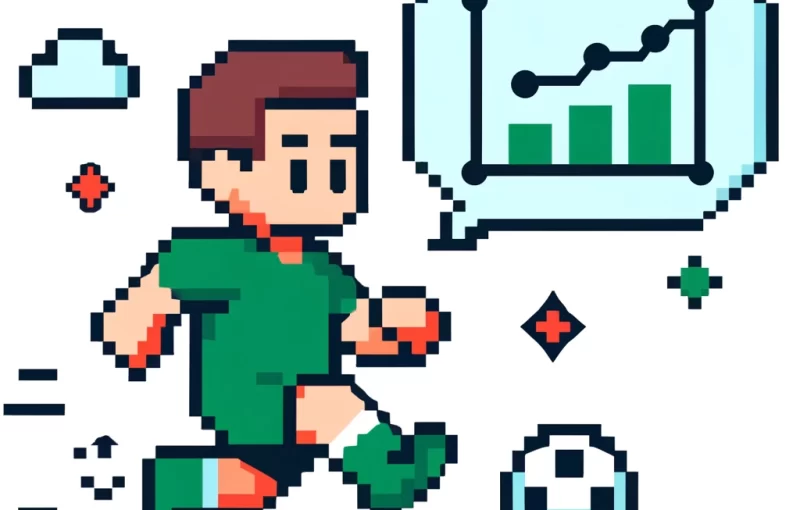
Predicting Player Performance
by Jon Scaccia July 3, 2024Predicting the future is no easy feat, especially when it comes to the dynamic and unpredictable world of soccer. Soccer fans and coaches alike have long sought ways to forecast player performance, and recent advances in artificial intelligence (AI) and deep learning are making this dream closer to reality.
In a groundbreaking study, researchers from Stanford University have developed a model that leverages convolutional neural networks (CNNs) to predict the performance of players in the English Premier League (EPL). This research has significant implications for soccer coaching and player development, offering a glimpse into the future of sports analytics.
The Challenge of Predicting Soccer Performance
Soccer is known for its high variability and low-scoring nature, making accurate predictions a complex task. Traditional methods have relied on historical data and basic statistical models, but these approaches often fall short due to the sport’s inherent unpredictability. The new model introduced by Daniel Frees, Pranav Ravella, and Charlie Zhang aims to tackle this challenge by employing advanced machine-learning techniques.
The researchers focused on predicting the Fantasy Premier League (FPL) points, a proxy for real-world player performance, using historical data from past game weeks. The FPL points system awards points based on various performance metrics, such as goals, assists, and clean sheets, making it a comprehensive measure of a player’s contributions on the field.
The Power of Convolutional Neural Networks
CNNs, typically used in image and video recognition, are particularly well-suited for this task due to their ability to identify complex patterns in data. The researchers compared their CNN-based model to other machine learning approaches, including Ridge regression and LightGBM, and found that the CNN outperformed these models, achieving better predictive accuracy with fewer input features.
The study revealed that recent FPL points, influence, creativity, threat, and playtime were critical factors in predicting future performance. By analyzing these metrics, the CNN model could forecast player performance more accurately than previous models. This advancement marks a significant step forward in the application of AI to soccer analytics.
Implications for Soccer Coaching and Player Development
The implications of this research extend far beyond fantasy sports. For soccer coaches and player development professionals, the ability to predict player performance can provide valuable insights into training and game strategies. By understanding which players are likely to perform well in upcoming matches, coaches can make more informed decisions about player selection and tactical adjustments.
Moreover, the model’s ability to analyze individual player performance metrics can help coaches identify areas for improvement and tailor training programs to address specific weaknesses. This personalized approach to player development can lead to more effective training regimens and ultimately improve team performance on the field.
Transfer Learning and Future Directions
The researchers also explored the potential of transfer learning, a technique that allows a model trained on one task to be adapted for another related task. They conducted experiments using soccer news data from The Guardian to predict player performance but found that the news corpus did not provide a strong predictive signal compared to the CNN model. However, this line of research opens up exciting possibilities for future studies, such as predicting win-loss odds for sports betting or developing AI agents for FPL management.
Conclusion
The study represents a significant milestone in the application of AI to sports analytics. By leveraging CNNs, they have developed a model that can accurately predict EPL player performance, offering valuable insights for coaches, players, and fantasy sports enthusiasts. As AI continues to evolve, we can expect even more sophisticated models that further enhance our understanding of the beautiful game.
Engage with Us
We’d love to hear your thoughts on this exciting development in soccer analytics! Here are a couple of questions to spark the conversation:
- How do you think AI can further revolutionize the world of sports analytics?
- What other sports do you think could benefit from similar predictive models?
Please share your comments below and join the discussion!
Step into the forefront of soccer innovation with ‘This Week in Soccer’.
Our newsletter is more than just words; it’s an interactive journey through the complexities of soccer analytics and strategy. Whether you’re a coach, educator, or enthusiast, our content is designed to spark curiosity and enhance your understanding. Subscribe today and be part of a community pushing the boundaries of soccer education.
About the Author
Jon Scaccia is a youth soccer coach and translational scientist. He has worked with the Exeter United Soccer Club for over five years, focusing on U5 development. In his day job, he works in data evaluation and implementation science.
Leave a Reply