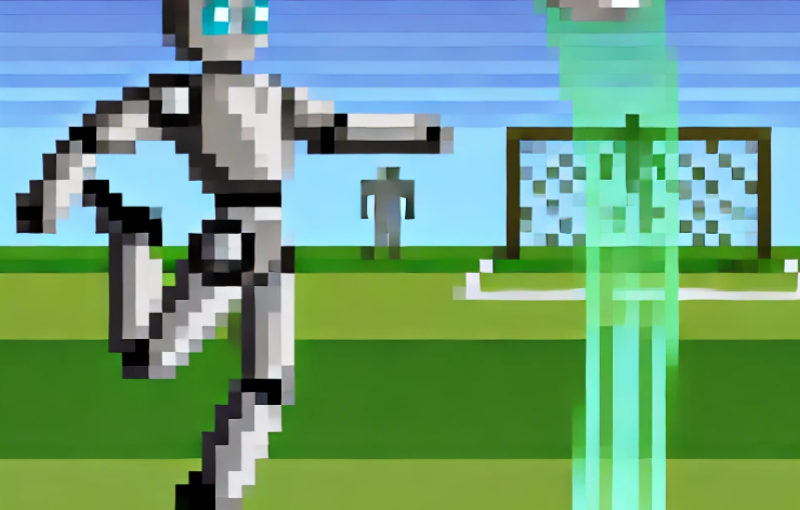
Predicting Soccer Performance with Machine Learning
by Jon Scaccia October 21, 2024Whether it’s how long to train or how hard to push players during practice, small changes can make a huge difference on game day. But how do coaches ensure their players are ready to give 100% without risking injury or burnout? The answer may lie in a surprising source: machine learning.
Yes, artificial intelligence, commonly associated with industries like tech or finance, is now making waves in the world of sports. New research is using machine learning to predict players’ perceived exertion levels—essentially, how hard they feel they’re working. These insights can help coaches fine-tune their training plans, keeping players in peak condition while minimizing the risk of overtraining.
Let’s dive into how this groundbreaking study works and what it could mean for soccer coaches everywhere.
The Hidden Data Behind Soccer Training
Think about a typical soccer practice: players are running, passing, shooting, and sprinting. While they’re moving, their bodies are also generating a mountain of data—everything from heart rate to the distance they cover. Traditionally, coaches would keep an eye on these metrics during practice, but there’s only so much information a person can process. This is where machine learning steps in.
Machine learning algorithms can analyze data faster and more thoroughly than any human coach. In this study, researchers gathered data from 26 professional soccer players over more than 5,400 training sessions and 732 matches. The data set included 174 different metrics, such as heart rate, speed, acceleration, and distance covered. All of this data was then used to predict one key metric: how hard players felt they were working, or their rating of perceived exertion (RPE).
RPE is a subjective score, with players rating how hard a session feels on a scale from 0 to 10. By using machine learning to predict RPE, coaches can get a clear picture of how training affects their players—without having to rely solely on players’ self-reports.
Why Predicting Exertion Matters
Why does RPE matter? Imagine this: two players both complete the same training session. One player might say the session was an “8” on the RPE scale, meaning they worked hard but could still push a bit more. The other player might rate it as a “5,” indicating it was relatively easy for them. By understanding these differences, coaches can adjust training to meet each player’s needs, making sure no one is pushed too far—or held back too much.
But what if a player’s RPE doesn’t match their performance metrics? Maybe a player reports a low RPE, but their heart rate data shows they were working much harder than they realize. This mismatch could be a red flag that the player is fatigued or hiding an injury. On the flip side, if a player reports a higher RPE than expected, it could indicate that they are struggling with their fitness and need to scale back.
Using machine learning to predict RPE based on objective data like heart rate and speed allows coaches to spot these discrepancies before they become serious problems. The result? Better training, fewer injuries, and peak performance on game day.
How Machine Learning Predicts RPE
In this study, the researchers compared nine different machine learning models to see which one did the best job predicting RPE. They also designed a deep learning architecture—essentially, a more advanced model that mimics the way the human brain processes information.
The standout performer? A tree-based machine learning model called ExtraTree. This model predicted RPE with a very low margin of error, making it highly reliable for coaches. What’s even better, it’s simple enough to run on almost any computer, meaning coaches don’t need access to supercomputers or fancy equipment to use it.
Key Findings from the Research
Several important insights emerged from the study:
- Maximum heart rate was the strongest predictor of RPE, meaning players’ heart rate during a session was closely linked to how hard they felt they were working.
- Other key factors included maximum acceleration and the total distance covered at moderate speeds (between 10-13 km/h). Together, these metrics helped the model predict RPE with high accuracy.
- The ExtraTree model performed well even when applied to new players who weren’t part of the original data set, showing that it’s versatile enough to be used across different teams.
By identifying these key factors, coaches can focus on the most important metrics during training sessions, cutting through the noise of hundreds of data points.
Applications for Coaches and Teams
How can coaches take these findings and apply them in the real world? Here are a few ways this study could change the way soccer teams train:
1. Personalized Training Plans
Machine learning models can help coaches tailor training sessions to individual players. If one player tends to report high RPE after moderate workouts, the coach can dial back their training load. Conversely, players with lower RPE scores might be ready to take on more challenging drills.
2. Injury Prevention
By closely monitoring discrepancies between predicted and reported RPE, coaches can spot signs of fatigue early. If a player’s predicted RPE is much lower than what they report, it could mean they’re at risk for injury. Catching this early allows for adjustments that could prevent injuries before they happen.
3. Better Game-Day Performance
When training is optimized using machine learning insights, players are less likely to experience burnout. They’re better prepared physically and mentally, leading to improved performance during matches. A well-rested player is a better player.
4. Data-Driven Decisions
Using machine learning, coaches can move away from gut feelings and make decisions based on hard data. This leads to more informed choices about when to push players harder and when to give them a break.
Applications: How to Use This Research
Coaches, teams, and sports scientists can implement these findings in several ways:
- Integrate machine learning models like ExtraTree into your training software to get real-time predictions of player exertion levels.
- Monitor key metrics such as heart rate and acceleration more closely, focusing on their relationship to perceived exertion.
- Use predicted RPE as a cross-check against players’ reported RPE to ensure they are training at the right intensity for their fitness levels.
- Adjust training loads based on the data, ensuring players are neither undertrained nor overtrained, reducing the risk of injury.
Conclusion
In the fast-paced, data-rich world of professional soccer, coaches need every advantage they can get. By using machine learning to predict players’ perceived exertion, they can fine-tune training, avoid injuries, and help players reach their full potential. As this research shows, the future of soccer training may lie not only in what happens on the field but in the power of data off the field.
Are you ready to delve deeper into the science and strategy of soccer?
This Week in Soccer offers you a unique blend of expert analysis, engaging infographics, and practical insights. Subscribe to our newsletter and stay ahead of the game with the latest in soccer analytics and coaching strategies. Make the move from spectator to strategist. Subscribe now!
Leave a Reply