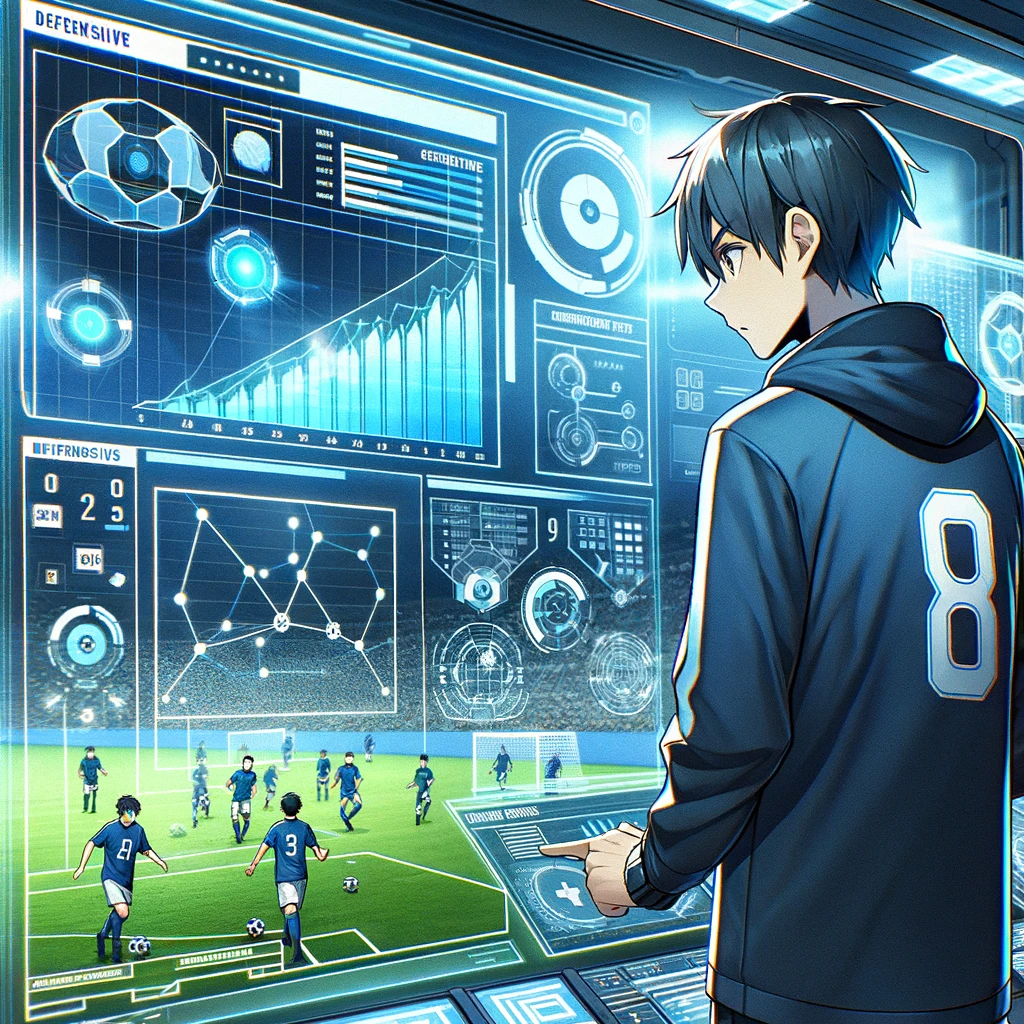
Enhancing Soccer Performance Analysis with AI and Machine Learning
In the dynamic world of soccer, understanding the nuances of player performance is crucial. The article from Nature, Context is key: normalization as a novel approach to sport specific preprocessing of KPI’s for match analysis in soccer, offers groundbreaking insights into how AI and machine learning can revolutionize soccer coaching and player development.
Unlocking the Potential of Data in Soccer
Like many other sports, soccer has seen a surge in the use of ‘Big Data.’ However, the sheer volume of data available has often led to confusion rather than clarity. As the study suggests, the key lies in contextualizing these data points. Putting data without context can lead to misleading interpretations.
Key Performance Indicators (KPIs) are widely used in sports analytics for various purposes, including player scouting, tactical analysis, and performance assessment. Traditionally, these KPIs have been raw data points, like the number of tackles or shots taken.
But this approach overlooks an essential factor: the context of the game.
The Role of AI and Machine Learning
The study highlights the integration of AI and machine learning in sports analytics. These technologies can process complex, non-linear relationships in sports data. However, they require well-defined input features to ensure accurate and interpretable results. This is where the concept of ‘normalization’ comes into play.
Normalization in soccer analytics is a powerful tool that adjusts data to reflect a team’s or player’s performance more accurately, taking into account the varying conditions of different matches. This process is particularly relevant when evaluating defensive actions, which factors like team possession can heavily influence.
Understanding Normalization in the Context of Soccer
The dynamics of a game change significantly based on which team has the ball. Teams with higher possession tend to have fewer defensive actions simply because they spend more time attacking. Conversely, teams with less possession are more often in defensive mode, leading to more defensive actions like tackles and interceptions.
This disparity creates a challenge: How do you compare the defensive performance of two teams with vastly different play styles? The answer lies in normalization. Analysts can level the playing field by normalizing defensive KPIs against team possession.
How Does Normalization Work?
Normalization works by adjusting the raw data to account for possession time. For instance, if Team A has a high possession rate, their number of tackles and interceptions might be lower, not necessarily indicating weaker defense, but simply less time spent defending. Conversely, Team B, with lower possession, might show more defensive actions.
To normalize this, analysts would adjust the defensive KPIs based on the proportion of time each team spends without the ball. This gives a more accurate representation of each team’s defensive effectiveness. In other words, it helps answer the question: “How well does a team defend relative to the amount of time it actually needs to?”
Implications for Soccer Coaching and Player Development
For coaches and player development staff, the implications of this approach are profound. Normalization provides a more accurate assessment of a player’s defensive abilities, independent of the team’s overall playing style. It allows coaches to identify which players excel in defensive actions relative to the time they spend defending.
This insight is crucial for talent scouting and developing training programs. Coaches can tailor their defensive drills and strategies to better understand each player’s strengths and weaknesses. Additionally, it can inform recruitment strategies by identifying players who excel in specific defensive areas that a team might lack.
Normalization also helps in evaluating the effectiveness of different tactical setups. For instance, a team switching to a more possession-based style can assess whether this change improves their defensive efficiency, using normalized KPIs as a benchmark.
Conclusion
The research presented in the Nature article emphasizes the need to integrate sport-specific knowledge into analyzing soccer performance data. By doing so, coaches and player development experts can gain deeper insights into the game, leading to more effective strategies and improved player performance. As the world of sports analytics continues to evolve, the incorporation of AI and machine learning, coupled with data normalization, will undoubtedly play a pivotal role in shaping the future of soccer.
Are you ready to delve deeper into the science and strategy of soccer?
This Week in Soccer offers you a unique blend of expert analysis, engaging infographics, and practical insights. Subscribe to our newsletter and stay ahead of the game with the latest in soccer analytics and coaching strategies. Make the move from spectator to strategist. Subscribe now!